Are Large Models in Industrial Manufacturing a Real Demand or Just Following the Trend?
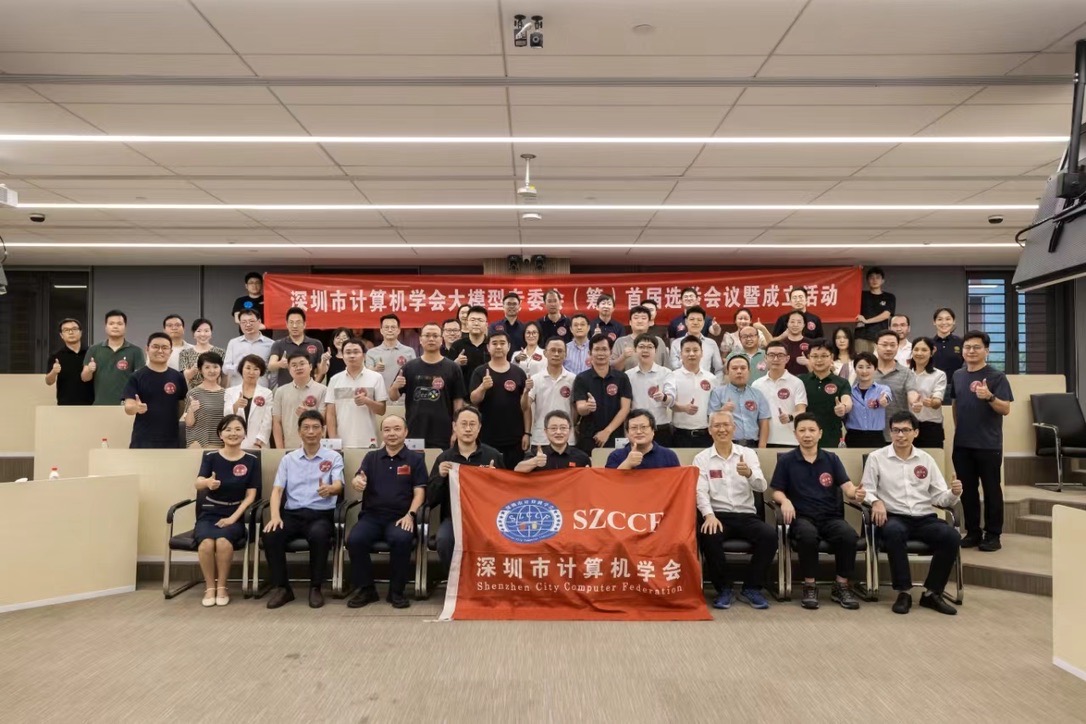
With the rapid advancement of AI technology, the wave of “large models” has inevitably reached the industrial manufacturing sector. These large models, which are complex AI systems trained on massive datasets, have demonstrated powerful capabilities across various domains, such as natural language processing, image recognition, and autonomous driving.
As the country with the most comprehensive range of industries, China is currently transitioning from digitalization to intelligent manufacturing. In this wave of digital transformation, large models hold great promise, with businesses widely hoping to leverage intelligent technologies to enhance production efficiency, optimize processes, reduce costs, and drive digital transformation forward.
However, while the vision is ambitious, the reality is somewhat less so. Unlike the heated competition in language models like ChatGPT, Baidu’s ERNIE Bot, or Alibaba’s Tongyi Qianwen, which are driving fierce price wars, the development of industrial large models has remained relatively quiet. The reason lies in the fact that industrial large models are still in their early stages and require further development and refinement.
On the afternoon of August 11, 2024, the Shenzhen Branch of the China Computer Federation’s Young Computer Scientists & Engineers Forum (CCF YOCSEF Shenzhen) hosted a viewpoint forum at the International Conference Center in Shenzhen University Town. The theme of the forum was “Are Large Models in Industrial Manufacturing a Real Demand or Just Following the Trend?”
Leanplans Participates as a Guest Moderator at the 2024 China Computer Federation Viewpoint Forum. The forum gathered over 80 attendees, including representatives from institutions such as Tsinghua Shenzhen International Graduate School, Peng Cheng Laboratory, and 48 companies including CGN, Leanplans Manufacturing, TCL CSOT, and Amazon.
Cognitive Understanding and Outlook
AI large models have already made significant strides across various industries. In 2022, DeepMind’s AlphaFold2 model successfully predicted nearly all known protein structures. More recently, the China Academy of Information and Communications Technology (CAICT) released the 2023 Large Model Application Casebook, selecting 52 exemplary cases of commercial large model deployments, covering sectors such as intelligent manufacturing, education, fintech, media, healthcare, and transportation.
During the introduction session, Professor Li Wenxian, Vice Dean of the Sino-German College of Intelligent Manufacturing at Shenzhen Technology University, used intelligent robotics as an example to explore the prospects and challenges of large models in driving industrial automation.
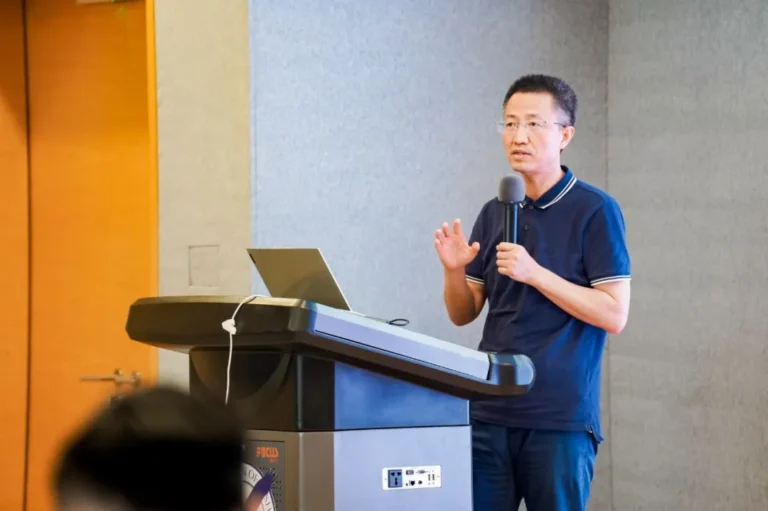
Professor Li highlighted that large models are currently being applied in manufacturing, logistics, and energy sectors. These applications include autonomous navigation and path planning, machine vision for quality inspection, and flexible assembly with collaborative robots.
When discussing the value of large model applications, Professor Li Wenxian identified three key areas of impact. First is efficiency improvement, where large models optimize production processes and increase equipment uptime. Second is enhanced product quality, achieved by detecting defects through automated systems to ensure consistency. Lastly, large models enable production flexibility, empowering businesses to quickly respond to market changes and meet demands for personalized and customized manufacturing.
Professor Li also pointed out several challenges currently faced by intelligent robots powered by large models, including data scarcity, high variability, quantifying uncertainty, safety assessments, and real-time performance issues.
He believes that future research should focus on optimizing computational efficiency, improving model generalization, and strengthening data security measures. Additionally, new ethical guidelines and regulations will need to be established to address the challenges posed by these emerging technologies.
While large models show significant promise, Liang Xiaojun, Director of the Industrial Intelligence Research Office at Peng Cheng Laboratory, issued a reminder that current industrial internet development remains focused on the network and platform layers, with insufficient progress in upgrading the underlying industrial control systems. He urged the industry to strengthen independent innovation and build a quality control system for the new industrial internet, ensuring sustainable growth in the sector.
The Symbiosis of Large and Small Models
Currently, due to the diversity of industrial scenarios, limitations in computing resources, the challenges of collecting and organizing training data in industrial fields, as well as concerns around the safety and reliability of large models, the application of large models in China’s industrial sector remains in an early exploratory phase.
Most existing applications of industrial large models are focused on knowledge-based Q&A systems. However, this basic functionality is far from sufficient. The true value of large models lies in their ability to penetrate deeper into the core execution processes of industry, which is currently the most pressing need in the manufacturing sector.
A fully realized industrial large model should cover all critical aspects of manufacturing, including solution planning, R&D design, experimental validation, production and manufacturing, operational management, and even marketing and after-sales services.
At present, large models struggle to comprehensively capture the characteristics and rules of specific industries or sectors, and they cannot fully meet the specific application needs of certain fields. To genuinely integrate large models into industrial applications, three core challenges must be addressed: first, the lack of deep understanding of the industry; second, insufficient familiarity with individual enterprises; and third, the issue of hallucination (i.e., generating incorrect, meaningless, or inaccurate outputs).
Overcoming these obstacles is essential for the deep application of large models in real industrial scenarios. Advancing the use of large models requires a gradual, step-by-step approach, with strategies that involve categorization, layering, and phased implementation. This process cannot be rushed.
During the debate session, Chengmin Tan, founder of Leanplans, served as the moderator, leading an in-depth discussion on the topic, “Which types of industrial enterprises need large model support?”
He pointed out that China currently has only 483,000 large-scale industrial enterprises, leaving approximately 4 million small and medium-sized factories facing significant challenges in digital transformation.
For these businesses, small models, due to their lightweight, efficient, and easy-to-deploy nature, often provide a quicker and more flexible solution to meet their practical needs. He further emphasized that while large models are well-suited for handling complex tasks, in many specific industrial scenarios, small models may actually be more appropriate.
For example, for small and micro enterprises with low production volumes and diverse product types, the flexibility and cost-effectiveness of small models are particularly attractive. According to a statistical analysis by the China Academy of Information and Communications Technology (CAICT) on 507 AI small model application cases, these models are predominantly applied in the manufacturing sector, accounting for as much as 57% of the use cases.
Looking ahead, it is expected that large models and small models will coexist in the industrial manufacturing domain for the long term, with their integration creating even greater value for enterprises.
On this topic, the panelists at the event engaged in in-depth discussions. They unanimously agreed that companies in the Greater Bay Area have a strong demand for advanced technologies, but when implementing them, they are more concerned with whether large models can reliably and effectively solve their pressing problems and the associated cost of investment.
Due to the varying challenges across different industries and sectors, large models need to be adapted to local conditions to overcome the “last mile” barrier from technology to practical application. This process can be accelerated through policy support and close collaboration between the tech sector and industry.
Currently, small models, with their efficiency, flexibility, and low resource consumption, continue to play an irreplaceable role in specific environments and tasks. Looking ahead, reducing the cost and technical barriers of deploying large models will be key to enabling these models to assist industrial enterprises in their upgrade and transformation efforts.